서 론
영양학적 가치와 섭취 편의성을 갖춘 새싹채소는 재배 기간이 짧고 성체 채소에 비해 비타민 C와 섬유질 등이 풍부한 영양 공급원으로 샐러드 형태로 간편하게 섭취하는 식품 중 하나이다(1). 우리나라에서는 10종 내외의 새싹채 소 작물이 재배·유통되고 있는데(2), 그 중 적콜라비는 양배 추와 순무의 교배로 만들어진 새로운 품종으로서 항암작용 과 항산화 작용에 효과를 가지는 것으로 알려진 글루코시놀 레이트, 안토시아닌, 카로티노이드와 같은 2차 대사산물을 함유하고 있다(3).
새싹채소의 경우, 별도의 가열 과정이 없이 섭취가 이루 어지기 때문에 생산단계에서부터 철저한 관리가 이루어져 위해요소로부터 안전한 새싹채소가 생산되어야 한다. 그러 나 국제적으로 새싹채소를 통한 식중독 세균에 의한 식중독 사고 피해 사례가 보고되고 있다(4,5). 새싹채소 매개 식중 독 사고는 1976년에 Bacillus cereus에 의한 첫 발생 보고 이 후, Salmonella spp., Escherichia coli O157:H7, Listeria monocytogenes 와 같은 식중독 세균이 원인이 되어 빈번하 게 발생되고 있다(6,7). 특히 2011년에는 E. coli O104:H4에 오염된 이집트산 호로파 종자를 이용한 독일 업체의 새싹채 소 재배·유통으로 인해 유럽국가 등 총 16개국에서 4,075명 의 식중독 환자가 발생하고 그 중 908명은 합병증으로 용혈 성요독증후군(hemolytic uremic syndrome)이 발생하였고 총 50명이 사망하는 사고가 발생한 바 있다(8,9). 식중독 세균이 새싹채소로 오염되는 경로는 농업용수, 작업자, 작 업도구, 오염된 종자 등인 것으로 알려져 있고, 식중독 사고 역학조사 결과에 따르면 오염된 종자가 가장 많은 비율로 보고되고 있어(10), 종자의 미생물학적 안전성이 중요하다. 새싹채소의 재배환경은 적당한 온도와 습도가 유지되어 세균이 생장하기에 적합한 것으로 알려져 있고(11), 종자 표면에 존재하는 E. coli O157:H7 등과 같은 식중독 세균이 새싹채소 종자가 발아한 이후 48시간 이내에 빠른 증식을 나타낸다고 보고되어 있다(12).
식중독 세균에 오염된 식품으로 인한 식중독 사고의 위 험성을 예측할 때에는 일반적으로 섭취할 당시의 식품에 존재하는 식중독 세균의 밀도에 따라 추정하게 된다(13). 생산에서부터 소비단계에 이르기까지 농식품에 존재하는 식중독 세균의 밀도변화를 예측하기 위해서 예측미생물학 (predictive microbiology)이 활용되고 있다(14). 그 중 미생 물 생장예측모델은 온도 등 미생물 생장에 영향을 미치는 변수를 기반으로 개발된 수학적 모델에 의해 주어진 시간의 미생물 밀도 변화를 예측하는 도구로 이용되고 있다(15). 국내외적으로 다양한 농식품의 식중독 세균에 대한 생장예 측모델이 개발되어 활용되고 있지만(16-18), 현재까지 새싹 채소를 대상으로 한 식중독 세균의 생장예측모델은 개발된 적이 없다.
따라서 본 연구는 국내 시판 새싹채소용 적콜라비 종자 에서 분리한 E. coli RC-4-D를 대상으로 새싹채소 재배 중 밀도를 예측할 수 있는 생장예측모델을 제시하기 위해 수행 되었다.
재료 및 방법
시중 유통되고 있는 새싹채소용 적콜라비 종자(원산지: 중국)를 구입하여 분석 대상 시료로 사용하였다. 적콜라비 종자 25 g을 샘플백(18×30 cm, Jinsung Uni-Tech, Goyang, Korea)에 담고 buffered peptone water(Oxoid, Basingstoke, Hampshire, UK)를 1:9(w/v)의 비율로 첨가한 뒤, 35℃ 배양 기에서 3시간 동안 배양한 후 배양액 1 mL을 9 mL의 EC broth, Reduced Blue Salts(Oxoid, Basingstoke, Hampshire, UK)에 넣고 44℃ 배양기에서 24시간 동안 배양하였다. 배 양 후 멸균 loop를 이용하여 배양액의 일부를 EMB(Oxoid, Basingstoke, Hampshire, UK) 배지에 옮긴 뒤 37℃에서 24시 간 동안 배양한 후 대장균으로 의심되는 형태의 콜로니를 nutrient agar(BD, Sparks, MD, USA)에 순수분리하였다. 분 리된 균주는 VITEX 2 System(VITEK®2, BioMerieux, Craponne, France)을 이용하여 생화학적 동정을 실시하였 다. 또한 균주의 16S rRNA 염기서열을 BLAST(Basic Local Alignment Search Tool)를 통해 염기서열 유사도가 높은 종을 확인하였다.
분리된 E. coli의 병원성 관련 유전자 보유 여부를 확인하 기 위해 specific primer를 이용한 multiplex PCR을 수행하였 다. 분리된 E. coli 균주의 chromosomal DNA 추출은 Genomic Plus DNA Prep Kit(Inclone, Yongin, Korea)를 이용 하였고, PowerChekTM Diarrheal E. coli 4-plex Detection Kit I(Kogene Bio-tech, Seoul, Korea)를 이용하여 shiga-like toxin 1과 2를 encoding하는 enterohemorrhagic E. coli의 병 원성 관련 유전자인 stx1, stx2와 enterotoxigenic E. coli의 병원성 관련 유전자인 heat-labile enterotoxin(LT), heat-stable enterotoxin(ST)의 보유 여부를 확인하였다. 추출한 genomic DNA를 template DNA로 사용하여 kit 제조사의 매뉴얼에 따라 primer Mix와 PCR premix를 micro-tube에 넣어 혼합한 다음 PCR을 진행하였다. PCR 조건은 Kit 제조사의 매뉴얼 에 따라 ① 95℃, 12분 → ② 95℃, 30초 → ③ 60℃, 45초 → ④ 72℃, 1분 → ⑤ 72℃, 10분으로 진행하였고 ②, ③, ④ 과정은 32 cycles 반복하였다. PCR 반응은 Thermocycler (C1000TM Thermal Cycler, Biorad, USA)를 이용하였다. PCR 반응 후, 증폭산물을 2% agarose gel을 이용해 전기영 동 후 병원성 관련 유전자 검출 여부를 확인하였다.
적콜라비에서 분리된 E. coli strain RC-4-D는 tryptic soy broth(BD, Sparks, MD, USA)에 배양한 뒤, 1 mL의 세균 배양액에 50% glycerol 0.25 mL을 섞어 -80℃의 초저온냉동 고에 보관하였다.
E. coli strain RC-4-D(wild type)를 rifampicin에 대한 내성 을 유도하기 위해 TSA 배지에 배양한 균주의 단일 콜로니 를 rifampicin(50 μg/mL) 항생제가 첨가되어 있는 tryptic soy broth에 접종한 뒤 37℃에서 24시간 동안 진탕배양하였 다. 배양 후 멸균된 loop를 이용하여 rifampicin(50 μg/mL)을 함유한 tryptic soy agar(BD, Sparks, MD, USA)에 계대한 후 37℃에서 24시간 동안 배양하며 자연발생 항생제 내성 균주가 배양되게 하였다. 이후 항생제가 첨가된 고체 배지 에 계대배양하여 항생제에 대한 내성을 가진 콜로니를 순수 분리하여 본 실험에 사용하였고 내성균주의 16S rRNA 염 기서열 분석 및 BLAST search를 통해 균주를 확인하였다.
rifampicin 내성을 가진 E. coli strain RC-4-D를 실험에 사용하였다. E. coli strain RC-4-D를 rifampicin이 포함된 3 mL의 tryptic soy borth에 접종한 뒤 37℃에서 24시간 동안 진탕배양하였다. 배지 성분 제거를 위해 배양된 세균 현탁 액을 4℃에서 4,000 rpm으로 10분간 원심분리하여 셀을 회수하였고, 회수된 셀은 3 mL의 멸균수로 2회 세척한 다음 최종적으로 3 mL의 멸균수에 재현탁한 뒤 실험에 사용하였 다.
시중 판매되는 적콜라비 종자(농우바이오, Suwon, Korea)를 구입하여 실험에 사용하였다. 90 g의 적콜라비 종자와 180 mL의 멸균수를 멸균 샘플백(WHIRL-PAK®, Fort Atkinson, WI, USA)에 넣고 104 CFU/mL의 밀도로 희석 한 E. coli strain RC-4-D 현탁액을 30 mL 접종한 다음 3분 동안 hand shaking법으로 균질화 하였다. E. coli strain RC-4-D이 접종된 적콜라비 종자는 25℃에서 6시간 동안 침종 과정을 거친 뒤, 상등 여액을 버리고 각 조사 온도 및 시간별로 종자를 2 mL 멸균수와 함께 페트리디쉬에 약 6 g씩 나누어 치상하여 10℃, 15℃, 20℃, 25℃, 30℃의 온도에 보관하면서 세균 밀도를 측정하였다.
각 페트리디쉬에 치상한 종자 또는 발아된 새싹을 한 페트리디쉬 내에서 2 g씩 3점의 시료로 subsampling한 후 E. coli strain RC-4-D의 밀도를 측정하였다. 2 g의 적콜라비 종자 또는 발아된 새싹을 취해 18 mL 멸균수를 담은 conical tube에 넣고 vortex를 이용하여 균질화하였다. 현탁액은 단 계희석하여 rifampicin이 첨가된 tryptic soy agar 배지에 도 말한 뒤 37℃에서 24시간 동안 배양한 후 밀도를 산정하였 다.
각 온도별(10, 15, 20, 25, 30℃)로 얻어진 세균 밀도를 엑셀 스프레드시트를 이용하여 log10 값, 평균, 표준편차 등으로 변환하였다. Baranyi model(Eq. 1-2)(19)을 이용하여 최대생장률[maximum growth rate, μmax(log CFU/h)]과 유 도기 [lag-phase duration, LPD(h)] 등 1차 kinetic parameter 값을 도출하였다.
Y0: 초기 세균 밀도(log CFU/g), Ymax: 최대 생장 세균 밀도(log CFU/g), Y(t): t시간에서 세균 밀도(log CFU/g), μ max: 최대생장률, h0: 미생물의 생리학적 상태
2차 생장예측모델은 suboptimal Ratkowsky square-root model(Eq. 3), suboptimal Huang square-root model(Eq. 4), Arrhenius-type model(Eq. 5) 등 3종류의 모델을 이용하여 적콜라비 종자에서 각각의 온도가 대장균의 생장에 미치는 영향을 설명하는 모델을 fitting 하였다.
a: 상수, T: 저장 온도(℃), T0: 최저 온도, Tmin: 세균 생장을 위한 이론적인 최저 온도
R: 기체상수(8.134 J/mol), ΔG′: 세균의 생장과 관련된 운동에너지, a, n: 계수, T: 온도(℃)
위와 같은 2차 생장예측모델은 IPMP(Integrated Pathogen Modeling Program) software(20)를 이용하였다.
2차 생장예측모델의 적합성을 평가하기 위해 통계학적 표준인 root mean squared error(RMSE), accuracy factor(Af), and bias factor(Bf)을 이용하여 검증하였다(21).
N: 생장 곡선에서 관측값의 수, P: 예측값, O: 관측값
결과 및 고찰
시중 유통되고 있는 적콜라비 종자(원산지: 중국산) 20점 의 시료 중 5점에서 E. coli를 분리하여 동정하였고, kit를 이용한 PCR법을 통해 1개 균주가 shiga-like toxin 2를 encoding하는 stx2 유전자를 보유하고 있는 것을 확인하였 다(data not shown). 해당 균주에 RC-4-D의 균주명을 부여하 였고 생장예측모델 개발에 사용하였다.
본 연구에서는 5개의 온도(10, 15, 20, 25, 30℃)에서 적콜 라비 종자에 접종한 E. coli strain RC-4-D의 시간 경과에 따른 밀도 변화를 조사하였다. Baranyi model을 적용한 적 콜라비 중 E. coli strain RC-4-D의 생장밀도곡선은 Fig. 1과 같다. 적콜라비 종자에서 E. coli strain RC-4-D의 초기 밀도 는 4.08-4.52 log CFU/g의 범위를 나타내었다. 10℃를 제외 한 4개의 모든 실험온도에서는 시간이 경과함에 따라 세균 의 밀도가 유의하게 증가하였으며, 온도가 상승함에 따라 밀도가 비교적 급격히 증가하는 경향을 나타낸다. 10℃의 경우에는 적콜라비 종자의 발아가 대부분 이루어지지 않고 일부만 발아를 하는 불균일한 발아율을 나타내었고(data not shown), E. coli strain RC-4-D를 접종한지 80시간 후에도 밀도가 유의하게 증가하지 않았다. 종자가 발아할 때 작물 로부터 분비되는 삼출물(exudates)이 세균의 밀도 증식에 큰 영향을 미친다는 사실이 알려져 있다(22). 또한 새싹채소 재배단계별 위생지표세균 밀도 및 유해미생물 오염도가 종자 단계보다 발아한 이후에 증가하는 양상을 나타내는 것으로 보고되어 있다(23). 따라서 종자의 발아가 E. coli strain RC-4-D 밀도 증가에 영향을 미치고 균일하게 발아를 하지 않은 10℃ 실험구에서는 유의한 밀도 증가가 이루어지 지 않은 것으로 판단된다.
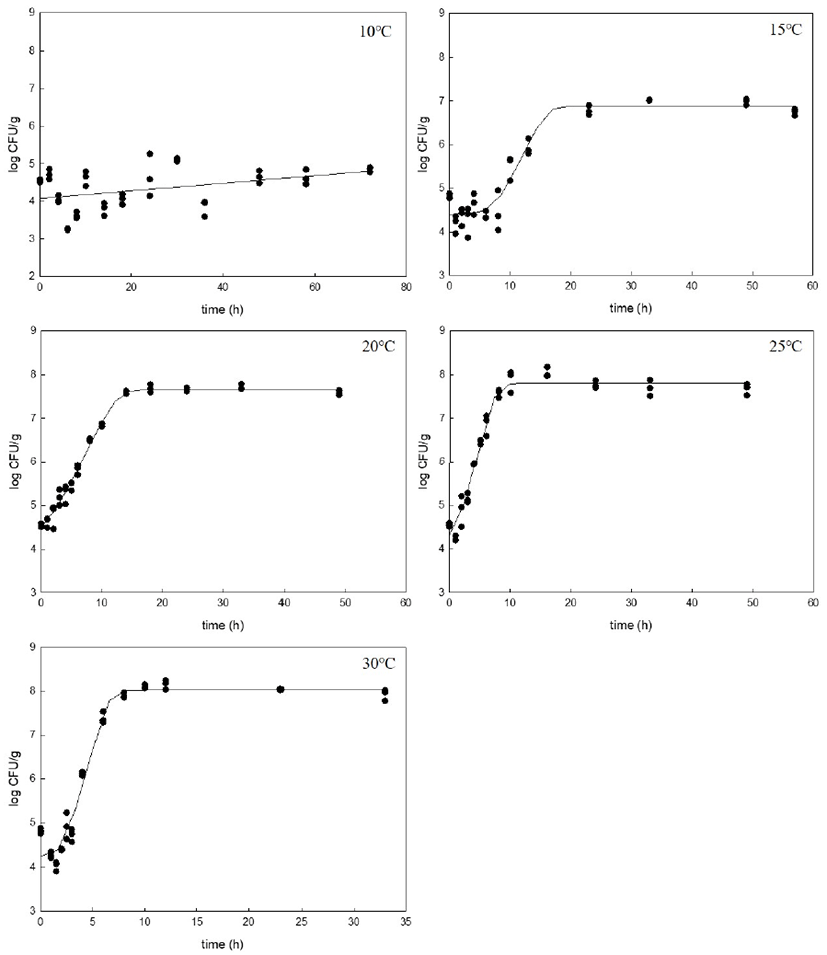
Baranyi model을 이용한 각 온도별 적콜라비의 E. coli strain RC-4-D의 생장밀도 fitting 결과를 바탕으로 최대생장 률(μmax), 유도기(LPD), 초기 세균 밀도(Y0), 최대 세균 밀도 (Ymax)를 도출하였다(Table 1). 최대생장률은 10℃에서는 0.01, 30℃에서는 0.87로 온도가 증가할수록 시간당 E. coli strain RC-4-D의 생장률이 증가하는 양상을 나타낸다. 이와 같은 양상은 외부온도가 적콜라비의 적정 생육온도와 세균 의 적정 생장온도에 가까워질수록 적콜라비 중 E. coli strain RC-4-D 증식 속도가 빨라진다는 것을 의미한다.
유도기(Lag-phase duration)의 경우, 유도기 값이 도출되 지 않은 10℃를 제외하고, 15℃에서 25℃까지 온도가 증가 할수록 유도기가 짧아지는 것을 확인하였다. E. coli는 일반 적으로 10℃에서 49℃까지 넓은 범위의 생육온도를 가지며 (24), 7.5℃ 이하의 저온이 되면 생장이 거의 이루어지지 않는 것으로 알려져 있다(25). 한편, 30℃에서는 20℃와 2 5℃에 비해 유도기가 길어지는 것을 확인하였다(Table 1). 이것은 30℃가 20℃와 25℃에 비해 E. coli의 생장에는 보다 유리한 온도이지만 적콜라비의 발아에는 불리한 온도이기 때문에 생장을 위한 유도기가 다소 길어지는 것으로 생각된 다. 이를 뒷받침할 수 있는 것으로서 Tokumasu 등(26)은 25℃에 비해 35℃에서 콜라비의 발아율이 다소 감소되는 것을 확인한 바 있다. 최대생장률과 유도기 모두 온도에 따른 유의한 차이가 있는 것으로 나타났고, 적콜라비 중 E. coli strain RC-4-D의 생장이 온도에 큰 영향을 받는 것을 확인하였다. 이러한 결과를 적콜라비 중 E. coli strain RC-4-D 2차 생장예측모델 개발에 아래와 같이 이용하였다.
Baranyi model을 이용한 1차 생장예측모델에서 도출된 온도별 최대생장률의 값을 이용해 Ratkowsky square-root, Huang square-root, Arrhenius-type models 등 3종류의 2차 생장예측모델 중 가장 적합한 모델 선발을 시도하였다. 각 모델별 2차 생장예측모델의 곡선은 Fig. 2와 같이 나타났으 며 각 모델의 매개변수 값은 Table 2와 같이 산출되었다. 모델에서 도출된 값과 같이 적콜라비 중 E. coli strain RC-4-D의 밀도 증식의 이론적인 최저 온도는 suboptimal Ratkowsky square-root model에서는 9.899℃로 suboptimal Huang square-root model에서는 9.997℃로 예측되었다 (Table 2). 이는 10℃에서는 적콜라비 중 E. coli strain RC-4-D의 유의한 밀도 증식이 이루어지지 않은 1차 생장예 측모델 검정 결과와 유사하게 예측되는 것으로 나타난다.
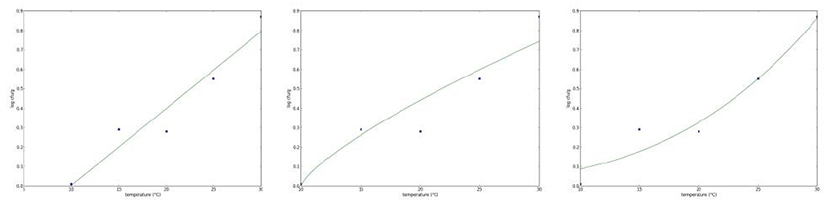
3종류의 2차 생장예측모델 중에서 온도가 적콜라비 중 E. coli strain RC-4-D의 생장에 미치는 영향을 가장 잘 설명 하는 모델인지를 평가하기 위해서 각 모델의 RMSE(root mean square error), Af(accuracy factor), Bf(bias factor) 값을 측정하였다. RMSE의 경우 관측값과 예측값의 차이로서 0에 가까울수록 적합한 모델로 평가되고 있는데(21), RMSE값이 suboptimal Ratkowsky square-root, suboptimal Huang square-root, suboptimal Arrhenius-type 모델에서 각 각 0.100, 0.123, 0.102로 나타났다. RMSE값에서는 suboptimal Ratkowsky square-root 모델이 관측값과 예측값 이 가장 근접한 것으로 나타났다(Table 2). Af의 경우, suboptimal Ratkowsky square-root, suboptimal Huang square-root, suboptimal Arrhenius-type 모델에서 각각 1.255, 1.223, 1.760으로 나타났다. 이것은 세 가지 모델의 예측값 이 관측값에 비해 각각 25.5%, 22.2%, 76.0% 다르다는 것을 의미한다. Af 값에서는 suboptimal Huang square-root 모델의 예측값의 정확도가 가장 높고 그 다음으로 suboptimal Ratkowsky square-root 모델이 높은 것으로 나타났고 suboptimal Arrhenius-type 모델은 정확도가 가장 낮은 것으 로 나타난다(Table 2). Bf는 suboptimal Ratkowsky square-root, suboptimal Huang square-root, suboptimal Arrhenius-type 모 델에서 각각 0.999, 1.075, 1.454로 예측되었다. Bf는 0.9에서 1.05 사이의 값을 적합한 것으로 평가하므로(21) 본 연구에 서는 suboptimal Ratkowsky square-root 모델이 적합한 것으 로 평가된다. 종합적으로 본 연구에 사용된 suboptimal Ratkowsky square-root, suboptimal Huang square-root, suboptimal Arrhenius-type의 3가지 모델 중 RMSE가 0.100, Af가 1.255, Bf가 0.999인 suboptimal Ratkowsky square-root model이 적콜라비 중 E. coli strain RC-4-D의 생장을 예측할 수 있는 가장 적합한 모델인 것으로 평가된다.
현재까지 국내에서는 주로 가공식품 위주로 미생물 생장 예측모델이 개발되었고 산업에 활용되어 왔다(27,28). 본 연구는 국내 새싹채소 재배용 종자에서 분리된 E. coli strain RC-4-D에 대한 생장예측모델 개발에 관한 것으로서 새싹 채소의 재배과정 중 E. coli의 밀도 변화를 정량적으로 예측 하고 위험성을 추정할 수 있는 기초자료로 활용될 것으로 판단된다. 또한 향후에도 지속적으로 농산물의 재배단계부 터 유통, 소비단계까지 미생물의 밀도를 예측할 수 있는 기술 개발을 활발히 진행하여 안전한 농산물 및 가공식품의 원료가 공급되는데 활용해야 할 것으로 생각된다.
요 약
본 연구는 시중 유통되고 있는 새싹채소 재배용 적콜라 비 종자에서 분리한 E. coli strain RC-4-D의 생장예측모델 을 개발하기 위해 수행되었다. 각 온도조건(10, 15, 20, 25, 30℃) 별로 적콜라비 중 E. coli strain RC-4-D 밀도 변화를 조사하였고 Baranyi model을 1차 생장예측모델로 이용하였 고 각 온도별로 최대생장률(μmax)과 10℃를 제외한 유도기 (LPD) 값을 도출하였다. E. coli strain RC-4-D의 최대생장률 에 대한 2차 생장예측모델로써 suboptimal Ratkowsky square-root, suboptimal Huang square-root, suboptimal Arrhenius-type 세 종류의 모델을 비교하였다. 모델 적합성 검정 결과, suboptimal Huang square-root 모델이 정확도가 가장 높고 suboptimal Ratkowsky square-root 모델이 편차가 가장 적은 것으로 나타났다. 종합적으로, RMSE가 0.100, Af가 1.255, Bf가 0.999인 suboptimal Ratkowsky square-root 모델이 온도의 영향을 설명하는 가장 적합한 2차 생장예측 모델인 것으로 나타났다. 본 연구에서 개발한 모델은 적콜 라비 새싹채소 생산에 있어서 E. coli의 생장을 예측하고 미생물 위해성평가를 수행하는데 활용될 것으로 기대된다.